
A paper authored by Warren B. Nelms Institute Associate Director, Dr. My T. Thai, and a team of researchers at University of Florida and New Jersey Institute of Technology (NJIT), has won Distinguished Paper Award at the Association for the Advancement of Artificial Intelligence (AAAI) 2023 Conference, held February 7-14, 2023.
The paper is titled “XRand: Differentially Private Defense against Explanation-Guided Attacks.”
The purpose of the AAAI conference series is to promote research in Artificial Intelligence (AI) and foster scientific exchange between researchers, practitioners, scientists, students, and engineers across the entirety of AI and its affiliated disciplines.
AAAI 2023 had 8,777 paper submissions. Of those, only 1,721 papers got accepted to the conference, and only 12 papers were selected for a Distinguished Paper Award.
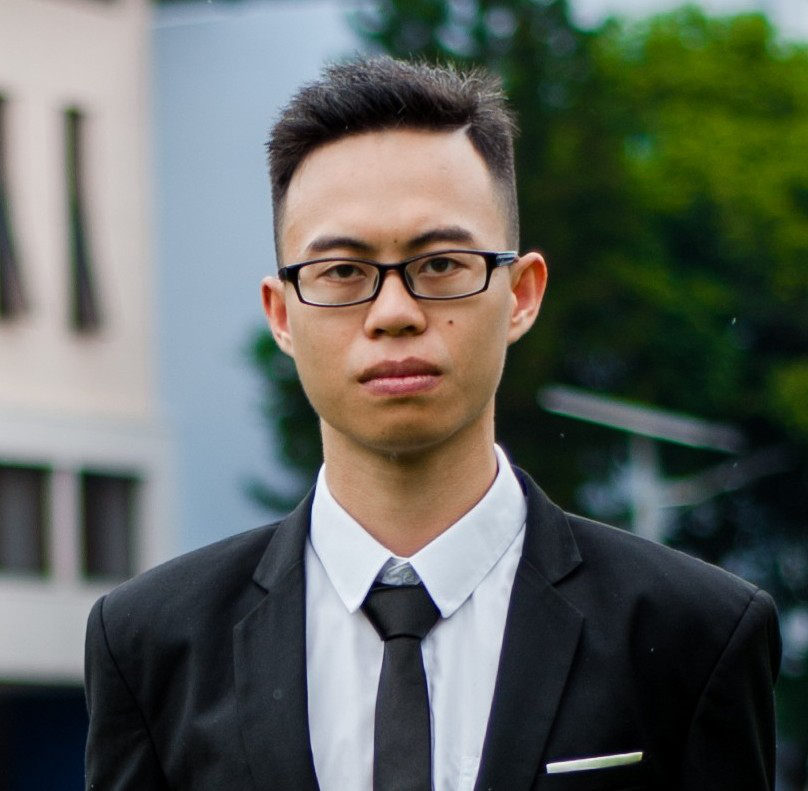
“Explainable AI is a double-edged sword; while it certainly helps black-box models become more interpretable, it also exposes their vulnerabilities. We are taking the first steps to tackle this trade-off with local differential privacy.”– Truc Nguyen, PhD Student, Lead Author
Paper Abstract:
Recent development in the field of explainable artificial intelligence (XAI) has helped improve trust in Machine-Learning-as-a-Service (MLaaS) systems, in which an explanation is provided together with the model prediction in response to each query. However, XAI also opens a door for adversaries to gain insights into the black-box models in MLaaS, thereby making the models more vulnerable to several attacks. For example, feature-based explanations (e.g., SHAP) could expose the top important features that a black-box model focuses on. Such disclosure has been exploited to craft effective backdoor triggers against malware classifiers. To address this trade-off, we introduce a new concept of achieving local differential privacy (LDP) in the explanations, and from that we establish a defense, called XRand, against such attacks. We show that our mechanism restricts the information that the adversary can learn about the top important features, while maintaining the faithfulness of the explanations.
Access the full paper here.